Predicting neutron experiments from first principles: A workflow powered by machine learning
E. Lindgren,
A. J. Jackson,
E. Fransson,
E. Berger,
S. Rudić,
G. Škoro,
R. Turanyi,
S. Mukhopadhyay,
and
P. Erhart
Journal of Materials Chemistry A ASAP
(2025)
arXiv:2504.19352
doi: 10.1039/D5TA03325J
zenodo: 15283533
(associated data)
Download PDF
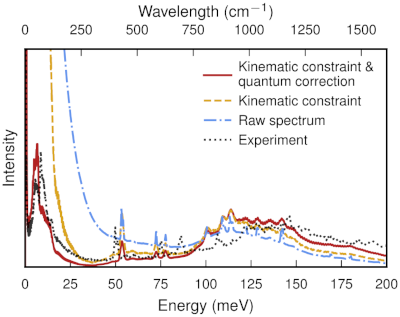
Machine learning has emerged as a powerful tool in materials discovery, enabling the rapid design of novel materials with tailored properties for countless applications, including in the context of energy and sustainability. To ensure the reliability of these methods, however, rigorous validation against experimental data is essential. Scattering techniques –using neutrons, X-rays, or electrons– offer a direct way to probe atomic-scale structure and dynamics, making them ideal for this purpose. In this work, we describe a computational workflow that bridges machine learning– based simulations with experimental validation. The workflow combines density functional theory, machine-learned interatomic potentials, molecular dynamics, and autocorrelation function analysis to simulate experimental signatures, with a focus on inelastic neutron scattering. We demonstrate the approach on three representative systems: crystalline silicon, crystalline benzene, and hydrogenated scandium-doped BaTiO3sub>, comparing the simulated spectra to measurements from four different neutron spectrometers. While our primary focus is inelastic neutron scattering, the workflow is readily extendable to other modalities, including diffraction and quasi-elastic scattering of neutrons, X-rays, and electrons. The good agreement between simulated and experimental results highlights the potential of this approach for guiding and interpreting experiments, while also pointing out areas for further improvement.