Tensorial properties via the neuroevolution potential framework: Fast simulation of infrared and Raman spectra
N. Xu,
P. Rosander,
C. Schäfer,
E. Lindgren,
N. Österbacka,
M. Fang,
W. Chen,
Y. He,
Z. Fan,
and
P. Erhart
Journal of Chemical Theory and Computation 20, 3273
(2024)
arXiv:2312.05233
doi: 10.1021/acs.jctc.3c01343
zenodo: 10257363,
10255268,
8337182
(associated data)
Download PDF
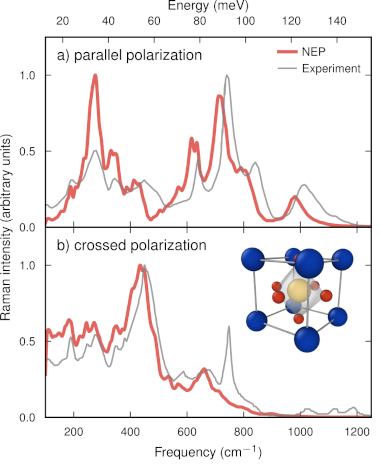
Infrared and Raman spectroscopy are widely used for the characterization of gases, liquids, and solids, as the spectra contain a wealth of information concerning in particular the dynamics of these systems. Atomic scale simulations can be used to predict such spectra but are often severely limited due to high computational cost or the need for strong approximations that limit application range and reliability. Here, we introduce a machine learning (ML) accelerated approach that addresses these shortcomings and provides a significant performance boost in terms of data and computational efficiency compared to earlier ML schemes. To this end, we generalize the neuroevolution potential approach to enable the prediction of rank one and two tensors to obtain the tensorial neuroevolution potential (TNEP) scheme. We apply the resulting framework to construct models for the dipole moment, polarizability, and susceptibility of molecules, liquids, and solids, and show that our approach compares favorably with several ML models from the literature with respect to accuracy and computational efficiency. Finally, we demonstrate the application of the TNEP approach to the prediction of infrared and Raman spectra of liquid water, a molecule (PTAF-), and a prototypical perovskite with strong anharmonicity (BaZrO3). The TNEP approach is implemented in the free and open source software package GPUMD, which makes this methodology readily available to the scientific community.