General-purpose machine-learned potential for 16 elemental metals and their alloys
K. Song,
R. Zhao,
J. Liu,
Y. Wang,
E. Lindgren,
Y. Wang,
S. Chen,
K. Xu,
T. Liang,
P. Ying,
N. Xu,
Z. Zhao,
J. Shi,
J. Wang,
S. Lyu,
Z. Zeng,
S. Liang,
H. Dong,
L. Sun,
Y. Chen,
Z. Zhang,
W. Guo,
P. Qian,
J. Sun,
P. Erhart,
T. Ala-Nissila,
Y. Su,
and
Z. Fan
Nature Communications 15, 10208
(2024)
arXiv:2311.04732
doi: 10.1038/s41467-024-54554-x
Download PDF
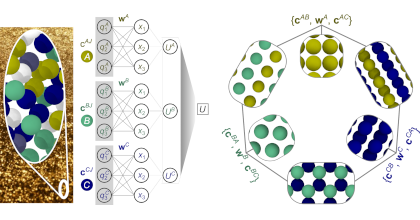
Machine-learned potentials (MLPs) have exhibited remarkable accuracy, yet the lack of general-purpose MLPs for a broad spectrum of elements and their alloys limits their applicability. Here, we present a promising approach for constructing a unified general-purpose MLP for numerous elements, demonstrated through a model (UNEP-v1) for 16 elemental metals and their alloys. To achieve a complete representation of the chemical space, we show, via principal component analysis and diverse test datasets, that employing one-component and two-component systems suffices. Our unified UNEP-v1 model exhibits superior performance across various physical properties compared to a widely used embedded-atom method potential, while maintaining remarkable efficiency. We demonstrate our approach’s effectiveness through reproducing experimentally observed chemical order and stable phases, and large-scale simulations of plasticity and primary radiation damage in MoTaVW alloys.